comparison of classification models for the stroke among elderly: A case study of Somdech Phra Pinklao Hospital
Keywords:
Stroke, Binary logistic regression, Decision trees, Imbalanced dataAbstract
The objective of this paper is to compare classification modes and study factors associated for stroke prediction in the elderly in Somdech Phra Pinklao Hospital, Bangkok, Thailand. The personal medical records of elderly patients who are over 60-year-old and visit the hospital in 2018, total 28,928 patients have been collected and preprocessed. Because of imbalance data, over-sampling technique is used to increase smaller group size. Then they have been partitioned into two groups. The former (80%) is used to construct models, which are the stepwise binary logistic regression (glm) and decision tree models (ID3, CART, J48, CTREE and C5.0) with Bootstrap Aggregating (Bagging). While the latter (20%) is used to evaluate the accuracy of the model. The result shows that the prevalence rate of stroke patients is 5.50% (95% CI 5.24% -5.76%). The most effective model is the C5.0 decision tree model with the accuracy of 95.31 percent, sensitivity of 94.48 percent, specificity of 96.12 percent, the positive prediction value of 95.93 percent and the negative prediction value of 94.73 percent. Using the C5.0 decision tree model, the important risk factors effecting on the stroke of the elderly by order are Transient ischemic attack, Age, Anemia, Epilepsy, Smoking, Clotting disorder and bleeding, Head injury, Heart disease, Cancer, Drinking alcohol, Kidney disease, The presence of implants and implants for the heart and blood vessels, Sex, Hypertension, Diabetes, Body mass index, Disorders of arteries, arterioles and capillaries, and Pulmonary embolism. While Overweight, obesity & hypernutrition and Metabolic disorder are not included the model to classify the stroke of the elderly.
References
International Health Policy Program (IHPP). The study of national burden of diseases and injuries among the Thai population in 2014. Nonthaburi: Graphico Systems; 2017.
Bureau of Non Communicable Diseases. Number and rate of patients in 2016 - 2018 (hypertension, diabetes, coronary heart disease, stroke, COPD); 2019. [Cites 16 June 2021]. Assessed from http://www.thaincd.com/2016/mission/documents-detail.php?id=13684&tid=32&gid=1-020
Strategy and Planning Division, Office of the Permanent Secretary Ministry of Public Health. Public Health Statistics A.D.2019. Nonthaburi: Strategy and Planning Division; 2020.
Zhuo Y, Wu J, Qu Y, Yu H, Huang X, Zee B, et al. Clinical risk factors associated with recurrence of ischemic stroke within two years: A cohort study. Medicine. 2020;99(26): e20830.
Muntham D, Ingsrisawang L. An Application of Decision Tree Algorithms for Diagnosis of the Respiratory System: A Case Study of Pranakorn Sri Ayudthaya Hospital. Journal of Health Systems Research. 2010;4(1):73-81.
Hongboonmee N, Trepanichkul P. Comparison of Data Classification Efficiency to Analyze Risk Factors that Affect the Occurrence of Hyperthyroid using Data Mining Techniques. Journal of Information Science and Technology. 2019; 9(1):41-51.
Thanathamathee P, Sirisathitkul Y. Improved Classification Techniques for Imbalanced Data Sets of Elderly’s Knee Osteoarthritis. Thai Science and Technology Journal. 2019;27(6):1164-1178.
Boonchuay K, Sinapiromsaran K, Lursinsap C. Minority split and gain ratio for a class imbalance. Proceeding of Eighth International Conference on Fuzzy Systems and Knowledge Discovery; 2011 july 26-28; Shanghai, China.
Chawla NV, Bowyer KW, Hall LO, Kegelmayer WP. SMOTE: Synthetic Minority Over- Sampling Technique. Journal of Artificial Intelligent Research. 2002;16:321-357.
Paranya P. Improving Decision Tree Technique in Imbalanced Data Sets Using SMOTE for Internet Addiction Disorder Data. Information Technology Journal. 2016;12(1):54-63.
Gosain A, Sardana S. Handling class imbalance problem using oversampling techniques: A review. Proceeding 2017 International Conference on Advances in Computing, Communications and Informatics. 2017 September 13-16; Udupi, India. IEEE Xplore; 2017.
Fernández A, García S, Herrera F, Chawla NV. SMOTE for Learning from Imbalanced Data: Progress and Challenges, Marking the 15-year Anniversary. Journal Of Artificial Intelligence Research. 2018;61:863-905.
RStudio Team. RStudio: Integrated Development for R. MA: RStudio, PBC; 2020. http://www.rstudio.com
Torgo L. Data Mining using R: learning with case studies, CRC Press; 2010.
Gareth J, Daniela W, Trevor H, Robert T. An introduction to statistical learning: with applications in R, Springer; 2013.
Kaiyawan Y. Principle and Using Logistic Regression Analysis for Research. Rajamangala University of Technology Srivijaya Research Journal. 2012;4(1):1-12.
Stevens J. Applied multivariate statistics for the social science. New Jersey: Lawrence Erlbaum Associate, Inc; 1996.
Quinlan R. Introduction of decision trees. Machine Learning. 1986;1(1): 81-106.
Quinlan JR. C4.5: Programs for Machine Learning. San Mateo, CA: Morgan Kaufmann; 1993.
Breiman L, Friedman JH, Olshen R, Stone CJ. Classification and Regression Trees. California: Wadsworth International Group; 1984.
Therneau T, Atkinson B, Ripley B. The rpart package. (Version 4.1-13) [Software]; 2018. https://cran.r-project.org/package=rpart
Hothorn T, Hornik K, Zeileis A. Unbiased recursive partitioning: A conditional inference framework. Journal of Computational and Graphical Statistics. 2006;15(3):651-674. https://doi.org/10.1180/106186006X133933.
Hothorn T, Seibold H, Zeileis A. Partykit: A toolkit for recursive partytioning. (Version 1.2-2) [Software]; 2018. https://CRAN.R-project.org/package=partykit.
Maung ETW, Aye ZM. Comparison of Data Mining Classification Algorithms, C5.0 and CART for Car Evaluation and Credit Card Information Datasets. National Journal of Parallel and Soft Computing. 2019;1(1):75-80.
Breiman L. Bagging Predictors. Machine Learning. 1996;24(2):123-140.
Upadhayay A, Shukla S, Kumar S. Empirical Comparison by data mining Classification algorithms (C 4.5 & C 5.0) for thyroid cancer data set, International Journal of Computer Science & Communication Networks. 2013;3(1):64-68.
Nilnate N. Risk Factors and Prevention of Stroke in Hypertensive Patients. Journal of The Royal Thai Army Nurses. 2019;20(2):51-57.
Reddy HP, Jaganath A, Nagaraj N, Visweswara RYJ. A study of age as a risk factor in ischemic stroke of elderly. International Journal of Research in Medical Sciences. 2019;7(5):1553-1557.
Yousufuddin M, Young N. Aging and ischemic stroke. AGING. 2019;11(9):2542-2544.
Zaorsky NG, Zhang Y, Tchelebi LT, Mackley HB, Chinchilli VM, Zacharia BE. Stroke among cancer patients. Nature communications. 2019;10(1):5172. https://doi.org/10.1038/s41467-019-13120-6
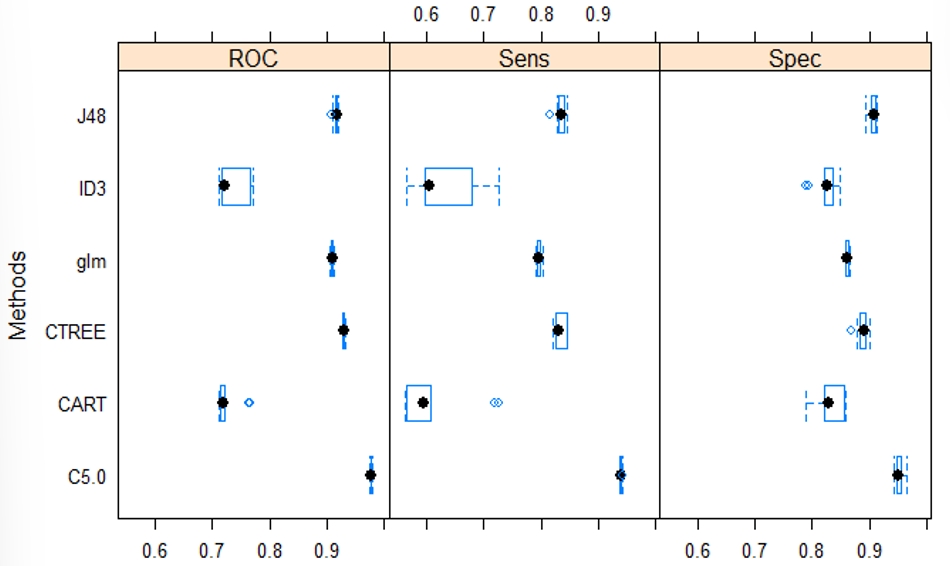
Downloads
Published
How to Cite
Issue
Section
License
Copyright (c) 2023 Naresuan Phayao Journal

This work is licensed under a Creative Commons Attribution-NonCommercial-NoDerivatives 4.0 International License.
ผู้นิพนธ์ต้องรับผิดชอบข้อความในบทนิพนธ์ของตน มหาวิทยาลัยพะเยาไม่จำเป็นต้องเห็นด้วยกับบทความที่ตีพิมพ์เสมอไป ผู้สนใจสามารถคัดลอก และนำไปใช้ได้ แต่จะต้องขออนุมัติเจ้าของ และได้รับการอนุมัติเป็นลายลักษณ์อักษรก่อน พร้อมกับมีการอ้างอิงและกล่าวคำขอบคุณให้ถูกต้องด้วย
The authors are themselves responsible for their contents. Signed articles may not always reflect the opinion of University of Phayao. The articles can be reproduced and reprinted, provided that permission is given by the authors and acknowledgement must be given.